AI Bias: When Antidiscrimination Laws Turn Sour
Women often get a raw deal from lenders. Banning gender data in machine learning can make things worse
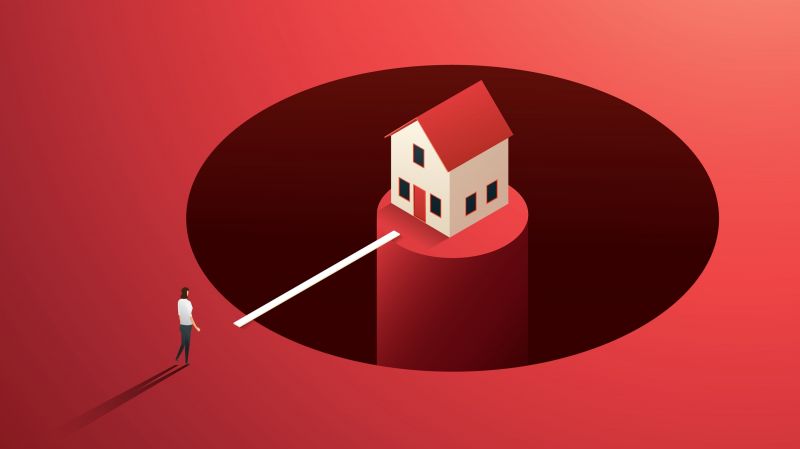
The consumer lending market can be a cold and confusing place. In the best of times, the loan approval process is opaque, and research suggests it’s stacked against certain groups of applicants—particularly women and other minorities. One recent study found that consumer loan applications submitted by women are 15 per cent less likely to be approved than those by men with the same credit profile.
Whether such seemingly unfair outcomes are due to bias or statistical anomaly is an open question. But the rise of fintech and algorithmic decision-making is pushing concerns to a new level. Fintech lenders use vast quantities of data of past borrowers to train machine learning models that can predict the odds of an applicant repaying or defaulting on a loan. If the data led to biased outcomes in the past, critics fear, won’t lending decisions guided by artificial intelligence just supersize the problem?
One possible response to such concerns is to simply ban lenders from using any information relating to the gender of loan applicants in their credit scoring calculations. Some governments agree, others do not. The U.S., for example, prohibits the collection and use of gender-related data in non-mortgage credit lending models. The European Union says yes to the collection of gender data but no to the use of that data at an individual level to train AI models. Singapore, on the other hand, allows the collection and use of gender data. (Countries such as Canada and Australia rely on case law and human rights declarations to help prevent discrimination in the provision of credit.)
A recently published study tests whether any of these approaches does a better job than others in reducing discriminatory lending outcomes. In the process, the study’s researchers ask the provocative question: Do the laws banning the use of gender information in assessing creditworthiness hurt rather than help the groups they are supposed to protect?
How was the study designed?
The researchers trained two types of models—machine learning and statistical (the prevailing non-AI approach)—on a large publicly available data set of more than 300,000 borrowers from a fintech firm. They simulated various antidiscrimination scenarios (with and without gender-related data) and measured their impacts on model quality and firm profitability. They used AI “explainability” methods and processes to understand what drives machine learning discrimination.
What did the study find?
- Prohibiting the use of gender-related data in credit scoring models (as is the approach in the U.S.) substantially increases discrimination and slightly decreases firm profitability.
- Allowing gender-related data to be collected (in the case of Singapore) allows lenders to improve their AI models and partially reduce gender discrimination while improving profitability.
- Machine learning models are less discriminatory, of better predictive quality and more profitable for fintech firms than non-AI statistical models.
What do I need to know?
The idea for the study came from conversations the researchers had with banking, insurance and fintech executives. The executives had observed that, in their use of AI-based systems, anti-discrimination laws often hurt, rather than helped, the groups they were supposed to protect.
This study put such observations to a rigorous test on a realistically large and publicly available dataset and found the executives were right: banning the collection and use of gender-related lending data in the name of anti-discrimination perversely increases discrimination against women. Lenders are also hurt since they are deprived of revenue from repayments that would have come from worthy applicants who are turned down.
But the study also shows that, if machine learning models are allowed to be trained with gender information, they can do a better job at mitigating discrimination against female loan applicants than the statistical models that non-fintech lenders generally use.
According to one of the study’s simulations, if lenders were able to use gender in their models, the rejection rates for women would have gone down by 30 to 50 per cent. In contrast, the rejection rates for men would have stayed constant.
How can this be? By design, AI models are trained to minimize total error, the study researchers explain, and therefore “tune more to relationships in the data that are reflective of the majority class.” In the case of consumer loans, men are the majority class. All else being equal, men tend to be less creditworthy than women but often come out stronger in other key attributes (such as work experience) that loom large in credit lending models. Therefore, when gender is excluded, so too are other variables that rely on the gender variable to provide predictive power to the model. Hence female loan applicants are unfairly turned down.
The researchers behind this study believe Singapore gets it right. If you want to legislate against discrimination in consumer lending, allow gender data to be collected so that AI models can be improved. “At the very least,” they write, “this would give firms the ability to assess the bias in their models, check whether their models do any harm and reduce discrimination by tweaking their approaches to sampling and modelling/data science techniques.”
But, they say, lenders must be responsible and transparent in how they collect and use that data and, most importantly, be held accountable for any damaging results. In conversations around the deployment of AI, this last part should not be overlooked.
Study Title: Antidiscrimination Laws, Artificial Intelligence, and Gender Bias: A Case Study in Nonmortgage Fintech Lending
Authors: Stephanie Kelley and Anton Ovchinnikov (Smith School of Business) and David R. Hardoon and Adrienne Heinrich (Artificial Intelligence and Innovation Center of Excellence, Union Bank of the Philippines)
Published: Manufacturing & Service Operations Management; early online publication.